[toc]html
Pandas 是基于NumPy 的一种工具,该工具是为了解决数据分析任务而建立的。 Pandas归入了大量库和一些标准的数据模型,提供了大量能使咱们快速便捷地处理数据的函数和方法。 主要包含两种数据类型:Series和DataFramemysql
相关帮助文档sql
示例代码以下数据库
from sqlalchemy import create_engine import pandas as pd username = '用户名' password = '密码' host = '链接地址' db = '数据库' port = 端口号 link = f'''mysql+pymysql://{username}:{password}@{host}:{port}/{db}?charset=utf8''' engine = create_engine(link, pool_recycle=3600)
核心方法read_sql数组
log:pd.DataFrame = pd.read_sql("SELECT * FROM log ORDER BY id DESC ",engine)
执行结果以下 app
import datetime log[log['create_time'] > '2020-01-15 16:14:22']
logs[['user_id','type']]
logs['type']
如今我须要将user_id对应的用户名找出来,示例代码以下dom
#查询出全部的用户,以便将log和users作join users:pd.DataFrame=pd.read_sql("SELECT * FROM users",engine) users
* users和log的字段太多,先作一下筛选函数
log=log[['type','user_id','project_id','create_time']] users=users[['id','username','real_name']]
执行join,使用merge方法,how指定左连,left_on指定左表使用的字段, right_on指定右表使用的字段工具
log.merge(users,how='left',left_on='user_id',right_on='id')
drop方法,axis为0表明行,1表明列spa
renameRes.drop('建立时间',axis=1)
dropRes.groupby(['type','real_name']).count()
groupby也能够能够传入一个可以访问索引上字段的函数
rng=pd.date_range('1/1/2020',periods=100,freq='D') ts=pd.Series(np.random.randn(len(rng)),index=rng) ts.groupby(lambda x:x.month).mean() 2020-01-31 0.182420 2020-02-29 0.200134 2020-03-31 -0.108818 2020-04-30 -0.187426 Freq: M, dtype: float64
by指定字段,ascending指定升序仍是降序
log.sort_values(by='user_id',ascending=False)
默认groupby后的结果是行索引是groupby的字段
log.merge(users,how='left',left_on='user_id',right_on='id').groupby('type').count()
groupby指定参数as_index
log.merge(users,how='left',left_on='user_id',right_on='id').groupby('type',as_index=False).count()
另外,还能够count完后直接调用reset_index方法
log.merge(users,how='left',left_on='user_id',right_on='id').groupby('type').count().reset_index()
log.T
使用rename方法,传递一个字典便可,以下
pd.DataFrame = res[['type','username','real_name','create_time']].rename({'create_time':'建立时间'},axis=1)
log['create_time'].astype(str)
count是必须依赖其余列作统计的,当只有一列的时候如何还使用count,是看不出统计字段的,正确的方法应该是使用size
test4=pd.read_sql("SELECT `type` FROM log LIMIT 100",engine) test4.groupby('type').size()
例如,要将create_time转为YY-MM-DD格式,可使用函数.dt.date
log['create_time'].dt.date
具体方法能够参考Series的API文档的Datetime操做
例如,转为大写
log['type'].str.upper()
具体方法能够参考Series的API文档的字符串操做
简单的理解就是一个更高级的groupby功能
df = pd.DataFrame({'foo': ['one', 'one', 'one', 'two', 'two', 'two'], 'bar': ['A', 'B', 'C', 'A', 'B', 'C'], 'baz': [1, 2, 3, 4, 5, 6], 'zoo': ['x', 'y', 'z', 'q', 'w', 't']}) df.pivot(index='foo', columns='bar', values='baz')
pivot_table支持分组后再聚合操做
df = pd.DataFrame({"A": ["foo", "foo", "foo", "foo", "foo", "bar", "bar", "bar", "bar"], "B": ["one", "one", "one", "two", "two", "one", "one", "two", "two"], "C": ["small", "large", "large", "small", "small", "large", "small", "small", "large"], "D": [1, 2, 2, 3, 3, 4, 5, 6, 7], "E": [2, 4, 5, 5, 6, 6, 8, 9, 9]} )
根据ABC分组,计算D的值,AB为行索引,C为列索引再使用sum函数,以下
df.pivot_table(values='D', index=['A', 'B'], columns=['C'], aggfunc=np.sum, fill_value=0)
通常使用matplotlib进行绘图 例如,统计全部的操做日志最多的前10个绘制直方图 先取出这些数据,以下
#获取全部操做类型最多的10条数据 countRes=log.groupby('type',as_index=False).count().drop(['create_time','project_id'],axis=1).rename({'user_id':'count'},axis=1).sort_values(by='count',ascending=False).head(10)
为了让图是递增的状态,咱们反转一下
countRes=countRes.iloc[::-1]
再使用matplotlib绘制直方图
import matplotlib.pyplot as plt plt.barh(countRes['type'],countRes['count'])
apply(DataFrame) DataFrame的函数,apply将函数应用到由各列或行所造成的一维数组上,默认是传入列的Series,能够指定axis=1传入行
applymap(DataFrame) DataFrame的函数,对每一个元素应用函数
map/apply(Series) 对应Dataframe的applymap
pands使用NaN(Not a Number)表示缺失数据,处理方法有
data = pd.Series(np.random.randn(10), index=[ ['a','a','a','b','b','b','c','c','d','d'], [1, 2, 3, 1, 2, 3, 1, 2, 2, 3] ] )
选取一个子集
data['b']
选取多个子集
data['b':'c']
选取内层:,号后面添加行号
data[:,2]
#Series转换为DataFrame data.unstack()
#将DataFrame转换为一个Series data.unstack().stack()
frame=pd.DataFrame(np.arange(12).reshape((4,3)), index=[ ['a','a','b','b'], [1,2,1,2] ], columns=[ ['Ohio','Ohio','Colorado'], ['Green','Red','Green'] ], )
frame.index.names=['key1','key2'] frame.columns.names=['state','color']
frame.swaplevel('key1','key2')
frame.sort_index(level=0,ascending=False)
df=pd.DataFrame({ 'a':range(7), 'b':range(7,0,-1), 'c':['one','one','one','two','two','two','two'], 'd':[0,1,2,0,1,2,3] }) df.set_index(['c','d'])
data=pd.DataFrame({ 'k1':['one']*3 + ['two']*4, 'kw':[1,1,2,3,3,4,4] })
data.drop_duplicates()
df = pd.DataFrame({'foo': ['one', 'one', 'one', 'two', 'two', 'two'], 'bar': ['A', 'B', 'C', 'A', 'B', 'C'], 'baz': [1, 2, 3, 4, 5, 6], 'zoo': ['x', 'y', 'z', 'q', 'w', 't']}) ```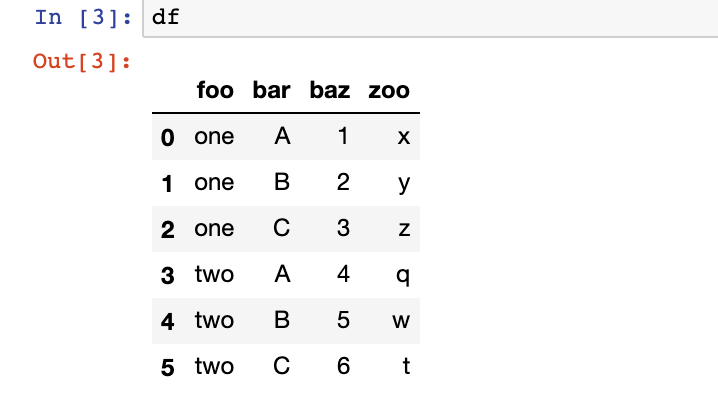 替换A和B为chenqionghe,第一个参数为查找值,第二个参数为替换的值
df.replace(['A','B'],'chenqionghe')
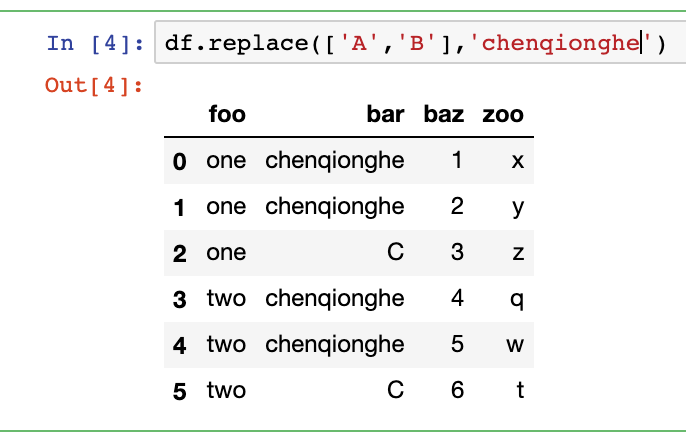 也能够传入字典,替换多个值
df.replace({'A':'cqh','B':'chenqionghe'})
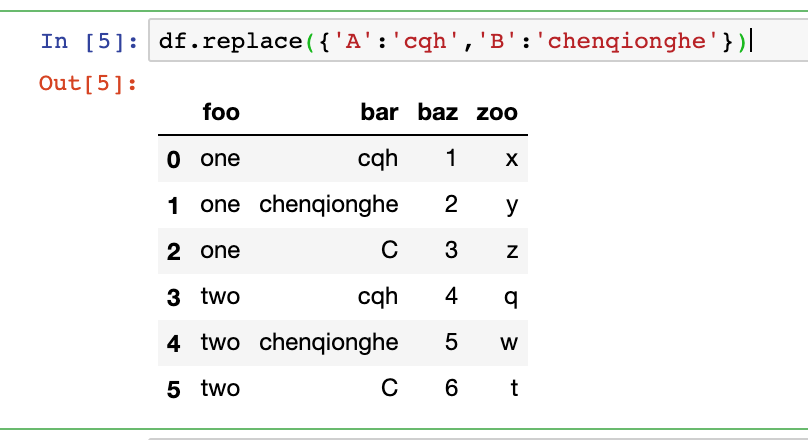 # 二11、如何链接两个dataframe-concat
df1=DataFrame(np.arange(6).reshape(3,2), index=list('abc'), columns=['one','two'] ) df2=DataFrame(5+np.arange(4).reshape(2,2), index=list('ac'), columns=['three','four'] )
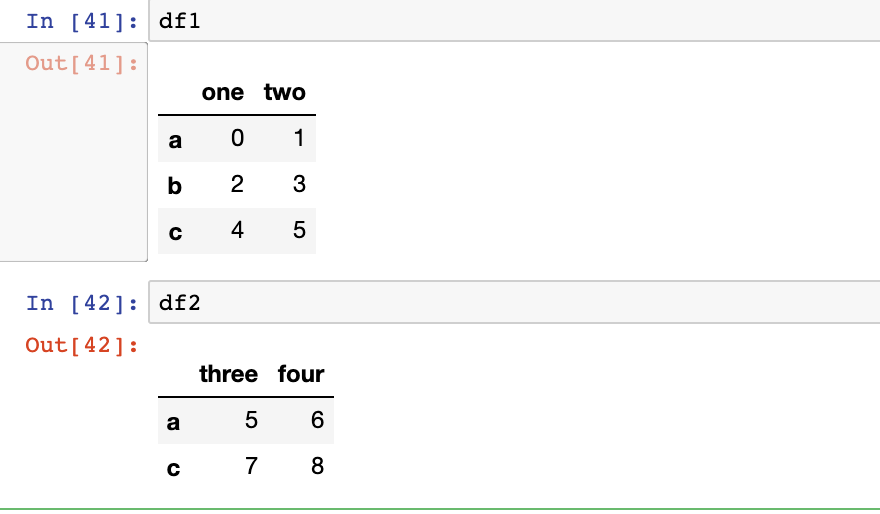 列拼接
pd.concat([df1,df2],sort=False)
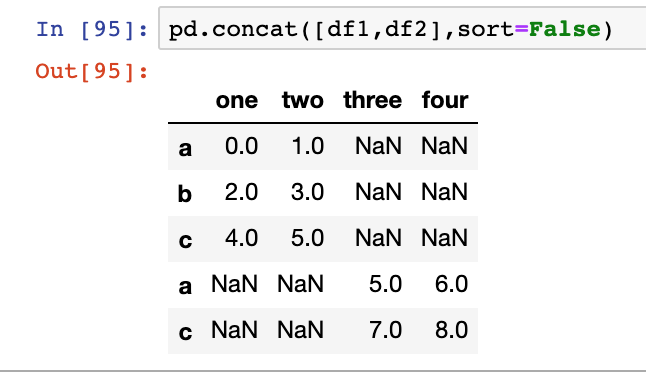 行拼接
pd.concat([df1,df2],axis=1,sort=False)
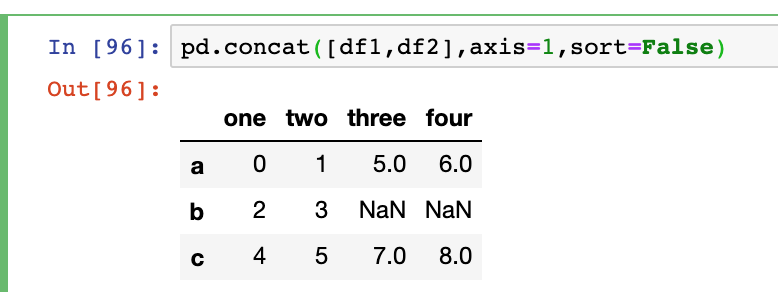 # 二12、如何从新设置索引-reindex
frame=pd.DataFrame(np.arange(9).reshape((3,3)), index=['a','b','c'], columns=['light','weight','baby'] )
#默认修改行索引 frame2=frame.reindex(['a','b','c','d'])
#同时修改行索引和列索引 frame.reindex(index=['a','b','c','d'],columns=['light','gym','muscle'])
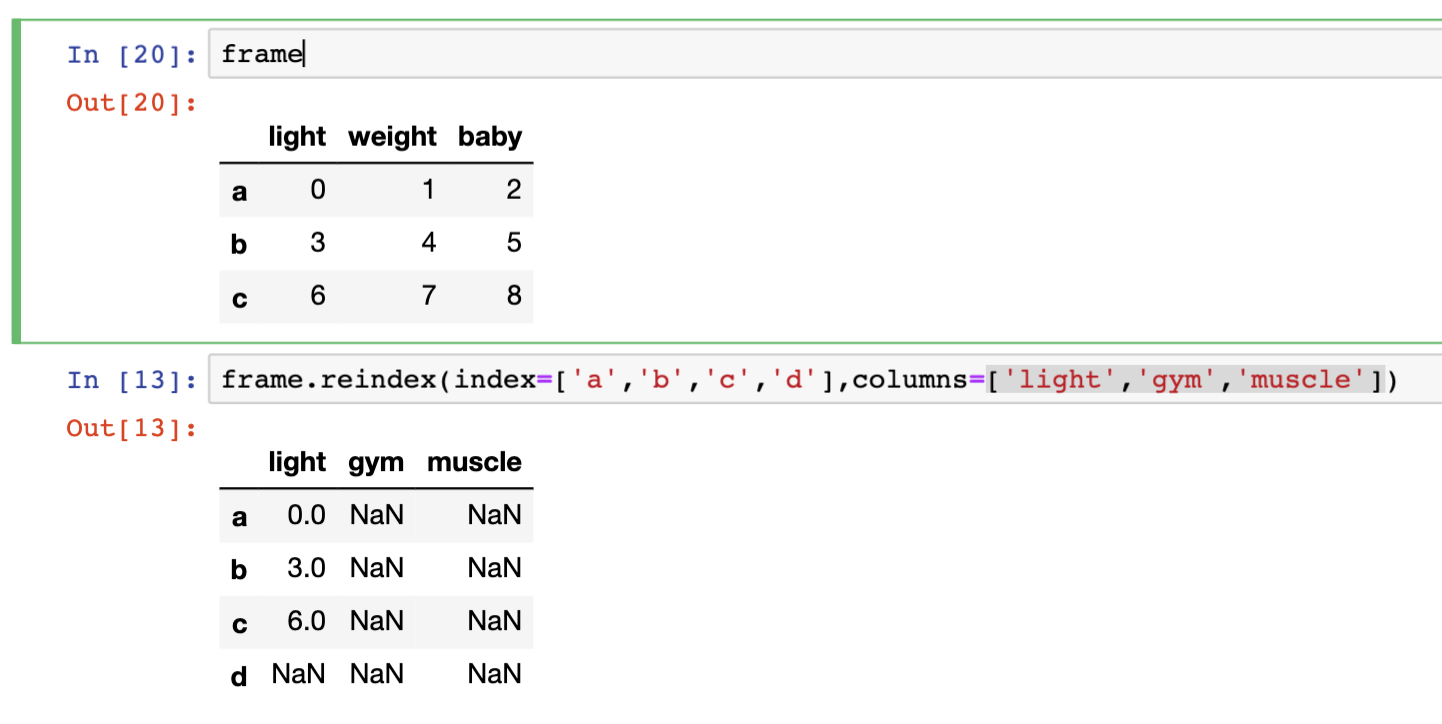 # 二12、如何从新采样-resample 建立3个周三的时间序列
ts1=pd.Series( np.random.randn(3), index=pd.date_range('2020-6-13',periods=3,freq='W-WED') )
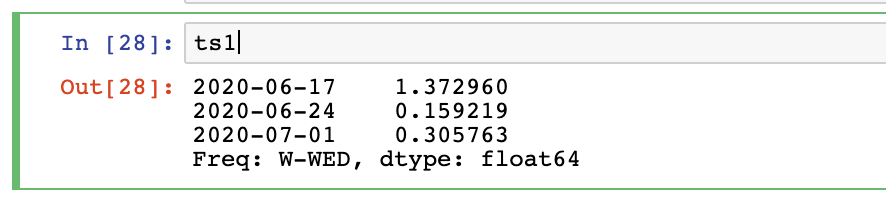 升采样转为每一个工做日
ts1.resample('B').asfreq()
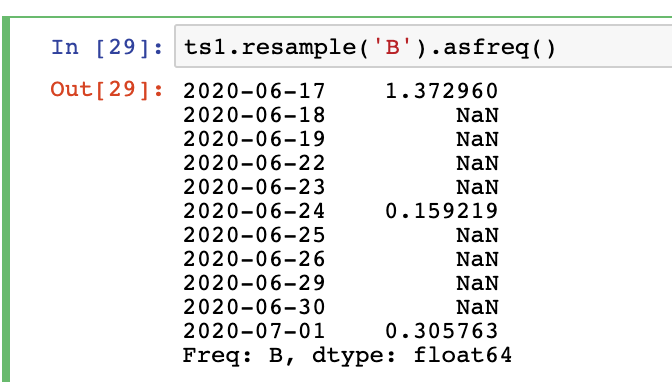 指定为ffill的填充
ts1.resample('B').ffill()
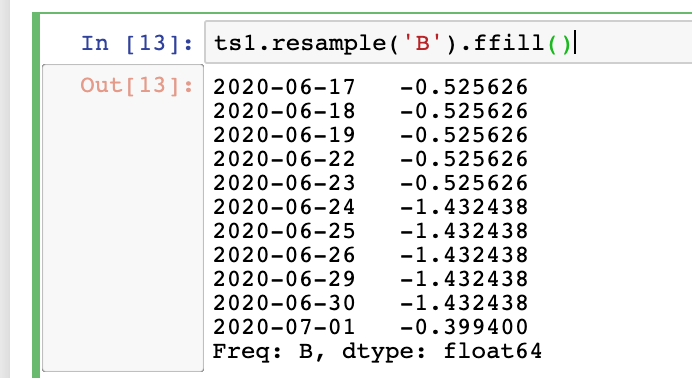 # 二十3、如何打补丁-combine_first combine_first至关于用参数对象中的数据为调用者对象的缺失数据”打补丁“
df1=pd.DataFrame({ 'a':[1,np.nan,5,np.nan], 'b':[np.nan,2,np.nan,6], 'c':range(2,18,4) }) df2=pd.DataFrame({ 'a':[5,4,np.nan,3,7], 'b':['chenqionghe',3,4,6,8] })
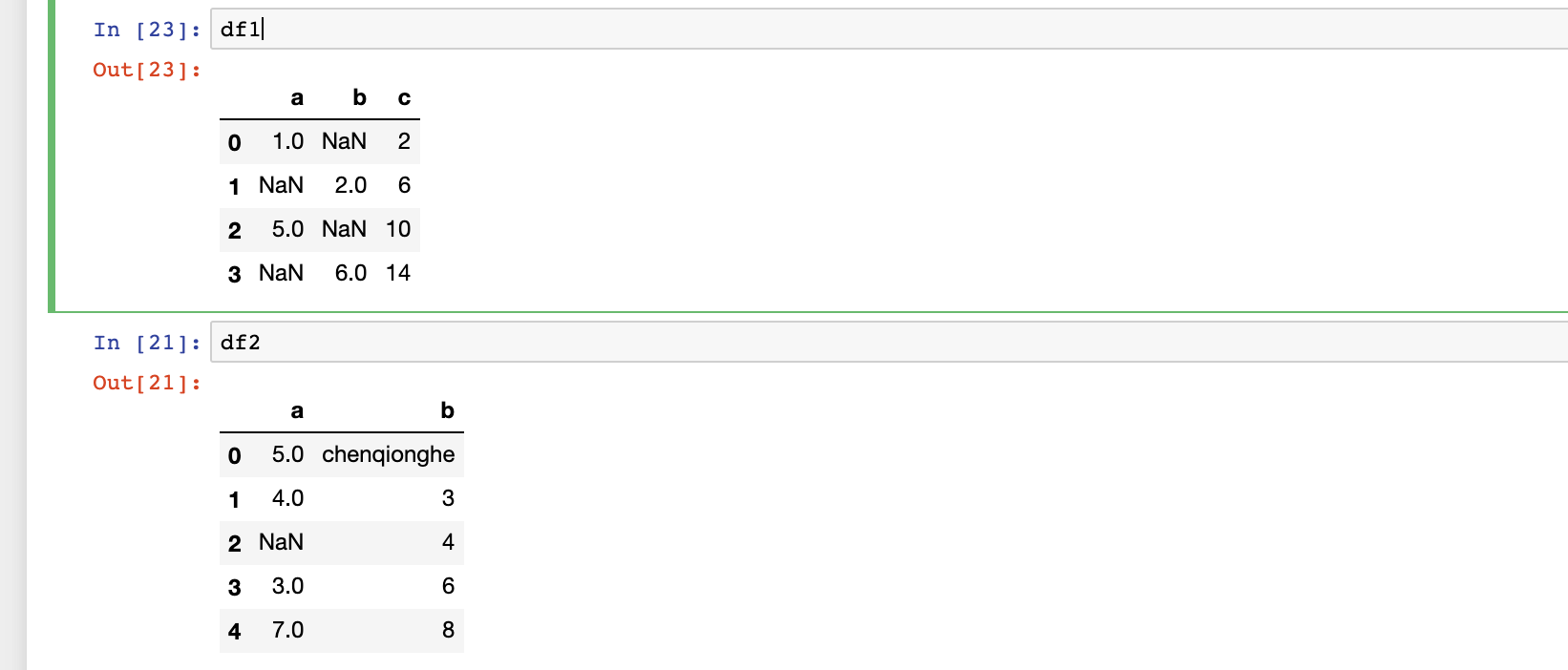 而后咱们能够用df2的值给d1打补丁,以下
df1.combine_first(df2)
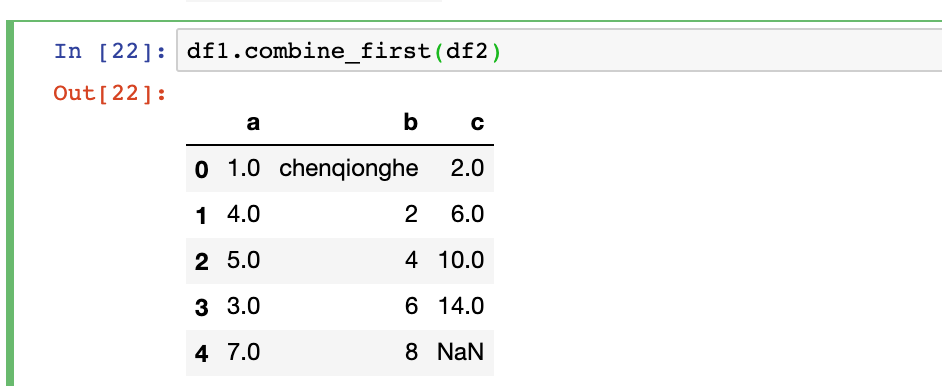 # 二十4、如何进行排名-rank
a=pd.DataFrame(np.arange(60).reshape(10,6),columns=['a','b','c','d','e','f'])
默认是对行进行排序,以下
a.rank()
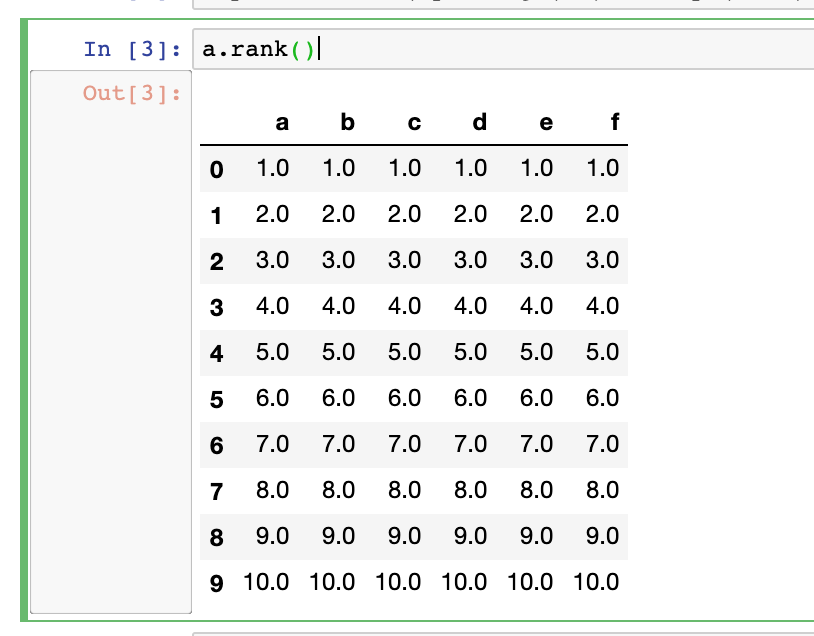 能够传axis=1对列进行排序
a.rank(axis=1)
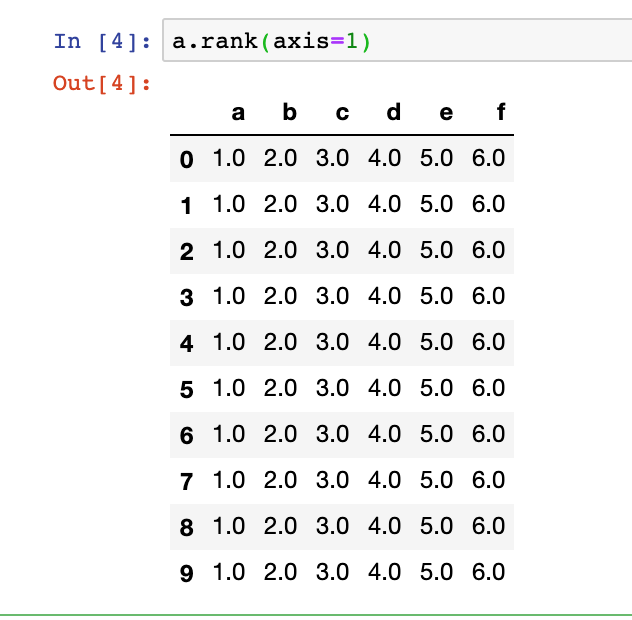 默认是升序,能够传入ascending=False进行降序
a.rank(ascending=False)
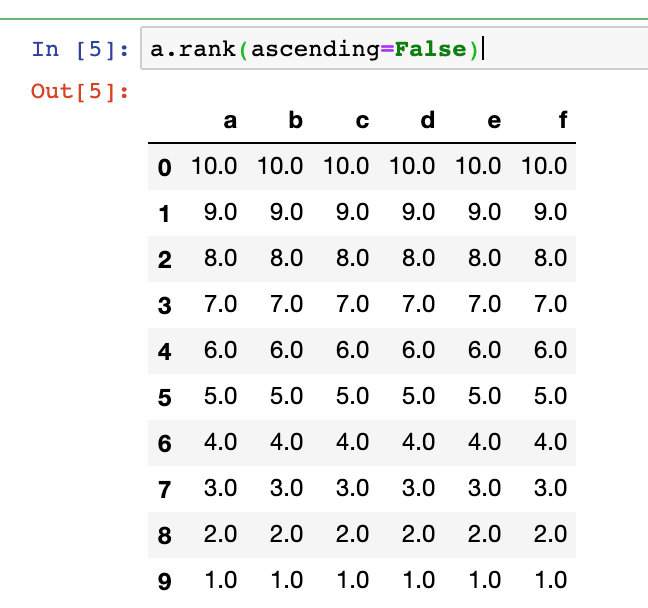 # 二十5、如何应用函数修改原dataframe-指定参数inplace pandas 中 inplace 参数在不少函数中都会有,它的做用是:是否在原对象基础上进行修改 - inplace = True:不建立新的对象,直接对原始对象进行修改; - inplace = False:对数据进行修改,建立并返回新的对象承载其修改结果。 默认是False,即建立新的对象进行修改,原对象不变,和深复制和浅复制有些相似。
原文出处:https://www.cnblogs.com/chenqionghe/p/12197607.html